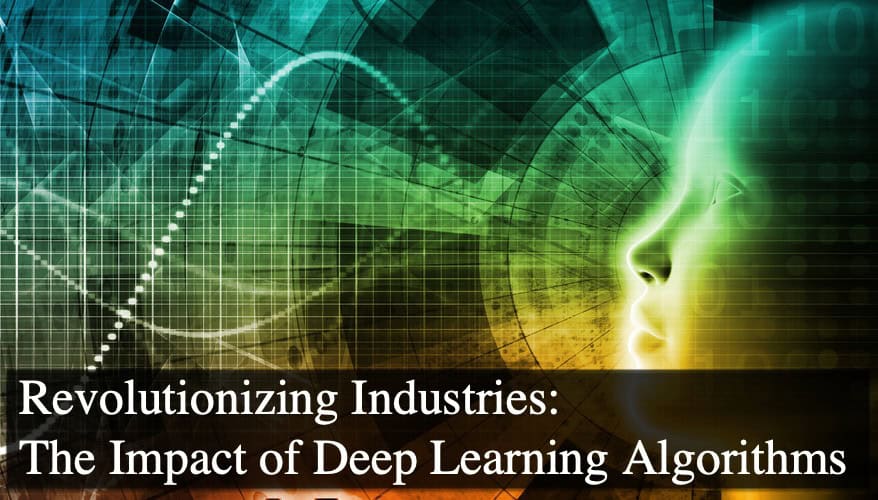
The rise of deep learning algorithms is transforming industries unprecedentedly, enhancing operational efficiency and enabling breakthroughs in healthcare, finance, and beyond. In healthcare, deep learning revolutionizes diagnostics and treatment plans, while in finance, it mitigates risks and predicts market trends with remarkable precision. These powerful algorithms, thriving on vast amounts of data, are just beginning to show their potential across various sectors, driving informed decision-making and setting the stage for the next wave of industrial evolution. Understanding these shifts offers insight into the future of technology and its vast applications.
Exploring the Core of Deep Learning
Deep learning algorithms are revolutionizing various sectors by using complex neural networks and advanced learning techniques. When combined with big data, these algorithms provide powerful insights and solutions.
Fundamentals of Neural Networks
At the heart of deep learning lies the neural network. Neural networks consist of layers of interconnected nodes or neurons resembling the human brain. Each neuron processes input and contributes to the output, creating a flow of information through the network.
Neural networks are composed of:
- Input Layer: Receives the raw data
- Hidden Layers: Perform computations and feature extraction
- Output Layer: Produces the final result or prediction
The interconnection and weight adjustment are crucial for the network’s learning ability. Training these networks with large datasets allows them to recognize patterns and make accurate predictions.
Advancements in Learning Algorithms
Significant advancements in learning algorithms have driven the progress of deep learning. Modern techniques such as Convolutional Neural Networks (CNNs), Recurrent Neural Networks (RNNs), and Transformer models enhance the network’s ability to process data types.
CNNs are widely used in image recognition because they capture spatial hierarchies. RNNs excel in sequence prediction and time-series analysis, making them ideal for applications like language modeling. Transformer models have revolutionized natural language processing, enabling more accurate translations and understanding of textual data.
These advanced algorithms enable neural networks to tackle more complex problems, enhancing the capabilities of deep learning systems.
Integrating Deep Learning with Big Data
Deep learning’s true potential is realized when integrated with big data. With the explosion of data in various forms, leveraging big data allows for deeper insights and more accurate predictions. This integration helps industries like healthcare, finance, and retail improve decision-making and operational efficiency.
Data preprocessing is essential to manage and clean massive datasets. Techniques like data augmentation and feature engineering also play a vital role in enhancing model performance. Utilizing distributed computing frameworks, such as Hadoop and Spark, allows for efficient handling and processing of vast data.
Organizations can drive innovation and transform their operations when deep learning and big data come together.
Deep Learning in Key Industries
Deep learning algorithms are transforming various sectors by offering precise insights, automating complex processes, and enhancing decision-making. This revolution brings unique advancements tailored to the specific needs of different industries.
Healthcare Revolution
Deep learning improves diagnostics and treatment in healthcare by analyzing complex medical data like MRI scans, X-rays, and pathology slides. Algorithms can detect diseases such as cancer early, providing physicians with crucial insights.
Wearable devices also benefit from deep learning by monitoring vital signs and predicting health issues before they become critical. Personalized treatment plans use patient data to tailor specific therapies, improving outcomes and reducing side effects. Deep learning thus fosters a more proactive and effective healthcare system.
Automotive Innovations
The automotive industry leverages deep learning to develop autonomous vehicles and advanced driver-assistance systems (ADAS). These algorithms process vast amounts of sensor data to navigate roads safely, recognize objects, and make real-time decisions.
Deep learning also enhances vehicle maintenance by predicting part failures before they happen, ensuring timely repairs. In-car entertainment and personalized driving experiences use data to adapt settings to your preferences. These innovations aim to make driving safer, more efficient, and enjoyable.
Financial Sector Transformation
Deep learning algorithms detect fraudulent activities in finance by analyzing transaction patterns and identifying anomalies. They provide more accurate credit scoring, swiftly evaluating various risk factors to offer consumers better financial products.
Deep learning benefits investment strategies by predicting market trends and optimizing portfolio management. Chatbots powered by these algorithms offer personalized customer service, handling queries and guiding financial decisions. This integration enhances the financial industry’s security, efficiency, and customer satisfaction.
Retail and E-Commerce Personalization
Retail and e-commerce companies use deep learning to personalize shopping experiences. Algorithms analyze customer behavior, preferences, and purchase history to recommend products that you are more likely to buy.
Inventory management uses these insights to predict demand, reducing overstock and stockouts. Visual search capabilities allow you to find products using images rather than text descriptions, enhancing the shopping experience. Deep learning thus drives customer engagement and operational efficiency.
Smart Manufacturing
Deep learning improves manufacturing production processes by optimizing supply chains and predicting equipment failures. Quality control systems use image recognition to identify product defects, ensuring higher standards and reducing waste. Additionally, deep learning for workplace safety is becoming increasingly crucial, as it can analyze data from various sensors to predict and prevent accidents, enhancing the overall safety of the work environment.
Robotic automation, powered by deep learning, adapts to changing conditions on the factory floor and performs tasks with precision. This technology boosts productivity, reduces downtime, and allows for more flexible manufacturing processes. Smart manufacturing drives efficiency and innovation in production environments.
Challenges and Considerations
Several critical aspects must be considered when dealing with deep learning algorithms. These include potential ethical implications, data privacy concerns, and the significant infrastructure requirements to support these technologies.
Ethical Implications
Implementing deep learning algorithms raises ethical questions related to bias and fairness. Algorithms trained on biased data can perpetuate or even amplify existing prejudices, leading to unfair outcomes for certain groups of people.
Furthermore, there’s the question of accountability. If an algorithm adversely affects individuals, it’s often unclear who should be held responsibleโ the developers, the users, or the organization deploying the technology. Ensuring transparency and fairness in these systems is crucial for maintaining public trust.
Data Privacy Concerns
Deep learning algorithms thrive on vast amounts of data, much of which can be personal and sensitive. Collecting, storing, and processing this data poses significant privacy risks. Unauthorized access or breaches can lead to misuse of personal information, a severe concern for individuals and organizations alike.
Moreover, regulatory environments such as GDPR in Europe and CCPA in California place strict requirements on handling personal data. Failing to comply with these regulations can result in hefty fines and damage to an organization’s reputation.
Infrastructure Requirements
Deep learning algorithms demand substantial computational resources. The training phase involves handling large datasets and performing complex calculations, which require high-performance hardware like GPUs and TPUs.
Additionally, maintaining this infrastructure can be costly in terms of hardware and energy consumption. Ensuring scalability and efficiency while managing these costs is a significant challenge organizations must address to harness deep learning technologies’ potential fully.
Conclusion
The transformative power of deep learning algorithms is revolutionizing industries by enhancing efficiency, enabling breakthroughs, and offering precise insights. From healthcare to finance and beyond, these algorithms drive innovation, improve decision-making, and set the stage for the next wave of industrial evolution. However, ethical considerations, data privacy concerns, and infrastructure requirements must be addressed to realize their full potential. Understanding and leveraging these advancements will shape the future of technology across various sectors.
Daniel Raymond, a project manager with over 20 years of experience, is the former CEO of a successful software company called Websystems. With a strong background in managing complex projects, he applied his expertise to develop AceProject.com and Bridge24.com, innovative project management tools designed to streamline processes and improve productivity. Throughout his career, Daniel has consistently demonstrated a commitment to excellence and a passion for empowering teams to achieve their goals.