The Importance of Cloud Computing for Scalable AI Edge Solutions
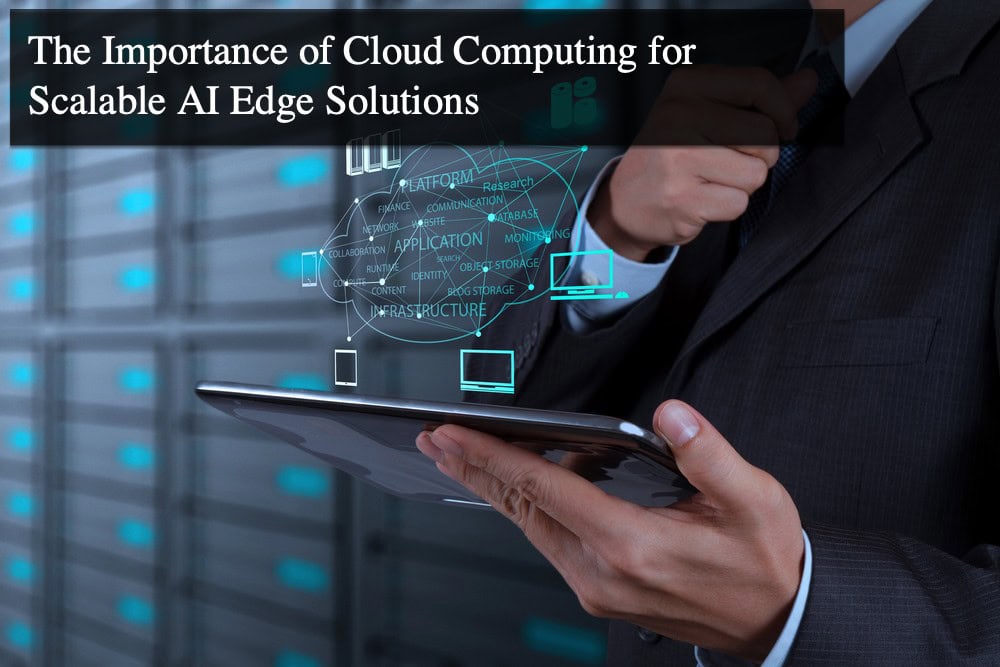
By facilitating scalable, real-time decision-making, cloud computing and edge artificial intelligence (Edge AI) revolutionize several sectors, including healthcare and manufacturing. Data management on edge devices, such as sensors or drones, is handled locally by AI, while cloud computing offers the infrastructure for training, improving, and scaling these models worldwide.
In this age of exponential data growth, this synergy represents more than just a technical breakthrough for companies seeking to remain competitive. The entire potential of Edge AI can only be realized with cloud computing; therefore, let’s talk about why.
What is cloud computing?
Cloud computing makes use of remote servers to store data and execute applications online. Companies may save money on hardware by using scalable resources provided by companies like Amazon, Google, and Microsoft. Businesses can manage large data volumes and complicated AI model training with little to no initial investment because of the model’s processing capability, affordability, and flexibility.
What Is Edge AI?
Edge AI enables the direct placement of AI algorithms onto neighboring sensors, as well as cameras and cellphones, instead of requiring permanent Internet connectivity. The system benefits offline areas and urgent situations by enabling instant data processing that ensures both performance speed and privacy protection.
By processing data at the source instead of moving huge amounts of data to the cloud, edge AI cuts down on internet use and speeds up response times. It is very useful in smart manufacturing, healthcare tracking, and self-driving cars because of.
Joining Cloud Computing with Edge AI
Integrating cloud and edge AI capabilities allows for the creation of lean and responsive systems. When it comes to building complicated AI models on enormous datasets, cloud computing provides scalable resources, whereas edge AI allows real-time data processing on local devices. When you combine the two, you can make decisions at the edge in real time, and cloud-based data and model tweaks make it possible for constant changes and improvements. We can make sure that AI systems can adapt to new data and changing customer needs by working together to speed up processing and cut down on lag time.
Cloud Powers Intensive AI Workloads
Modern cloud infrastructure provides the high-performance computing necessary for training complex AI models. Leveraging advanced hardware like H200 GPUs, cloud platforms can dramatically shorten training cycles, often delivering results up to ten times faster than traditional on-premise systems. This speed is crucial for sectors such as finance, healthcare, and autonomous driving, where AI must process vast datasets and adapt rapidly to maintain accuracy in real time.
Seamless Scalability for Growing Demands
Scalability is one of the cloud’s strongest advantages. When data loads surge, cloud environments enable organizations to instantly boost computational capacity without performance bottlenecks. With globally distributed data centers, edge devices can receive updated models and sufficient processing support as needed, all without requiring major infrastructure overhauls.
Flexibility and adaptability
Cloud computing is flexible because it can handle software updates and connect to many IoT devices without any problems. Delivering cloud modernization services that fit the ever-changing demands of AI infrastructure requires this level of flexibility. Before releasing the best-performing AI model to edge devices, developers may test out new models in the cloud.
The main advantages are:
- Integrated model validation before edge rollout
- Simple ability to update or revert AI features
- Quick and painless data and system integration with external sources.
Using this method, systems may be adjusted to meet the evolving needs of consumers and adapt to new technologies.
Using cloud optimization services from Tech-Stack helps businesses optimize their cloud-edge hybrid infrastructure by decreasing latency, managing cost efficiency, and improving resource usage across dispersed networks. For more info, go to https://tech-stack.com/services/cloud-optimization.
Confidentiality and Safety
Linking cloud and edge computing improves security. By handling personal data locally, Edge AI lessens the risk of security breaches while in transit. Cloud service companies, on the other hand, spend money on safety features like firewalls, encryption, and tight authorization rules. Businesses and groups that need to follow rules like GDPR and HIPAA and deal with private information should use this multi-layered security option.
Minimization of Waste and Expenses
Running complex AI models on every edge device will be an expensive ordeal. By using complicated calculations, cloud computing is able to lower these expenditures. For the simple reason that we only upload the most crucial files to the cloud. This reduces the amount of high-capacity hardware components needed for each edge device and saves bandwidth. It turns out that this hybrid method really cuts down on energy and money use.
Protecting Data: A Joint Obligation of the Cloud and the Edge
The use of AI enhances privacy by allowing sensitive data, such as patient vitals, to remain on-device. Still, the cloud offers extra security. Cloud computing gives you access to features like military-grade security and the freedom to follow rules like GDPR and HIPAA. For secure auditing and storage, healthcare wearables need the cloud, even though they do most of their work locally. Federated learning is a game-changer since it allows edge devices to do model training locally. With a fair balance between privacy and collective intelligence, only model changes are sent to the cloud, rather than raw data.
Suggested articles: The Role of Cloud-Based Infrastructure in Project Management | 10 Best Practices for Success In Cloud Automation Testing