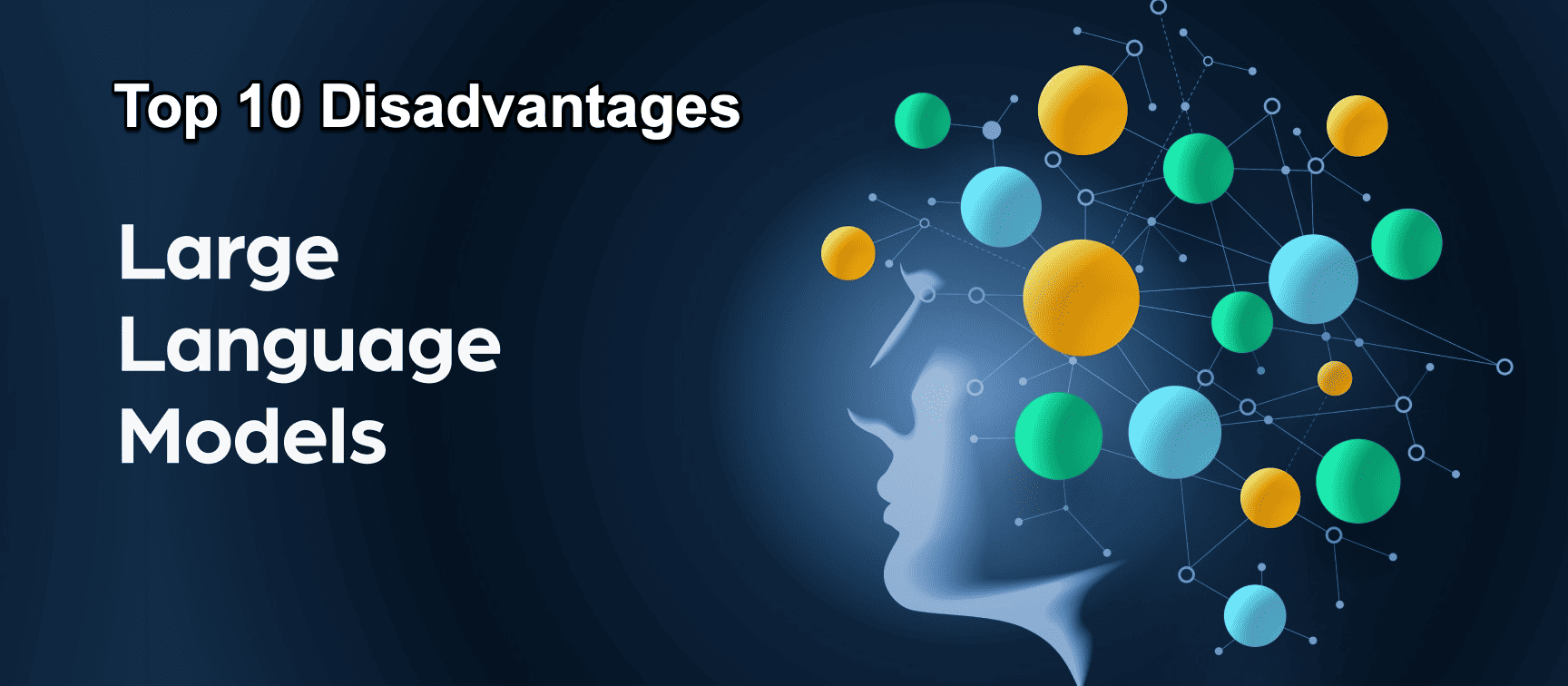
In technological advancements, Large Language Models (LLM) have emerged as a revolutionary tool, reshaping how we interact with information and digital interfaces. These sophisticated algorithms, trained on vast datasets, can understand and generate human-like text, offering unprecedented automation, creativity, and information processing opportunities. However, like any groundbreaking technology, LLM comes with its own set of challenges and limitations. Understanding these drawbacks is crucial for navigating the ethical, practical, and technical landscapes that surround their use.
LLMs, by their very nature, are complex and resource-intensive. They require significant computational power and large datasets to train, often leading to substantial energy consumption and environmental impacts. The reliance on extensive data also raises concerns about privacy and the potential for perpetuating biases present in the training data. Furthermore, the ability of LLMs to generate convincing text can be a double-edged sword, leading to issues like misinformation, loss of jobs in certain sectors, and challenges in content moderation. As we delve into the nuances of these models, it’s essential to critically examine their capabilities and limitations to harness their potential responsibly.
Top 10 Cons & Disadvantages of Large Language Models (LLM)
Three primary concerns stand out when discussing the cons of Large Language Models: ethical implications, accuracy and reliability issues, and the environmental impact. Ethical concerns arise from the potential misuse of these models for generating fake news, deepfakes, or aiding unethical activities due to their advanced text generation capabilities. Accuracy and reliability issues are paramount, as these models can sometimes produce incorrect or biased information influenced by the data they were trained on. Lastly, due to the massive computational resources required for their operation, the environmental impact of LLMs raises significant sustainability questions.
1. Ethical Implications
The ethical implications of LLMs are a significant concern, especially considering their ability to generate realistic and persuasive text. This capability can be exploited to create misleading information or deepfakes, contributing to the spread of misinformation. For instance, in a real-life scenario, an LLM generated fake news articles indistinguishable from authentic news, causing public confusion and mistrust. This example underscores the potential for LLMs to be used maliciously, making it challenging to discern truth from fiction. The lack of accountability in using these models further complicates the ethical landscape. As LLMs become more sophisticated, the line between real and artificial content blurs, raising questions about authenticity, trust, and the integrity of information.
2. Accuracy and Reliability
Despite their advanced capabilities, LLMs can struggle with accuracy and reliability. They sometimes generate factually incorrect or contextually inappropriate content from limitations in their training data or algorithms. A notable example was when an LLM provided incorrect medical advice in a public forum, leading to potential health risks for individuals who might follow such guidance. This incident highlights the risk of relying on LLM-generated content for critical decision-making. The challenge lies in ensuring that these models are technically proficient but also reliable and safe for various applications, from healthcare to legal advice.
3. Environmental Impact
The environmental impact of LLMs is a growing concern. The energy consumption required to train and operate these models is substantial, contributing to carbon emissions and environmental degradation. A case in point is the training of a state-of-the-art LLM, estimated to emit as much carbon as five cars over their entire lifetimes. This footprint is alarming, considering the increasing use of such models across industries, from content creation to enhancing support chat functionalities. The demand for more data and complex algorithms only exacerbates this issue, raising questions about the sustainability of LLMs in their current form.
4. Bias and Representation
Bias in LLMs is a critical issue. These models often reflect and amplify biases in their training data, leading to unfair or discriminatory outcomes. For example, an LLM was found to exhibit racial and gender biases in its language generation, reinforcing harmful stereotypes. This issue is particularly concerning in applications like hiring or law enforcement, where biased algorithms can potentially affect individuals’ lives. The challenge is to develop methods to mitigate these biases, ensuring that LLMs are fair and representative of diverse perspectives.
5. Job Displacement
The automation capabilities of LLMs pose a threat to certain job sectors. Their ability to perform tasks traditionally done by humans, such as writing, customer service, and even coding, can lead to job displacement. A real-life instance of this was observed in the journalism industry, where an LLM was used to write articles, reducing the need for human writers. While LLMs can increase efficiency and reduce costs, they also raise concerns about the future of work and the need for new skill sets and job roles in the evolving digital economy.
6. Dependence on Data Quality
LLMs’ effectiveness is heavily dependent on the quality of their training data. The model’s output will likely inherit these issues if the data is flawed, incomplete, or biased. A real-life example occurred when an LLM generated inaccurate historical information due to the limited and biased historical data it was trained on. This reliance on data quality challenges ensuring that LLMs are well-rounded, accurate, and unbiased in their responses, especially in fields where precision is crucial.
7. Security Risks
Security is a significant concern with LLMs. Their ability to process and generate sensitive information can be exploited for malicious purposes, such as phishing or creating convincing spam messages. This became evident when an LLM was used to craft sophisticated phishing emails that successfully bypassed traditional security filters. This example highlights the need for robust security measures to protect against the misuse of LLMs and safeguard sensitive data.
8. Overreliance and Skill Degradation
An overreliance on LLMs can lead to skill degradation in critical thinking and writing. As people become more dependent on automated tools for content creation, there’s a risk of diminishing their abilities to analyze, write, and think critically. This was observed in educational settings, where students increasingly relied on LLMs for writing essays, potentially impairing their learning and writing skills. Balancing the use of LLMs while maintaining and developing essential human skills is a challenge that needs addressing as these models become more ingrained in our daily lives.
9. Lack of Transparency and Explainability
LLMs often operate as “black boxes,” with limited transparency and explainability regarding how they arrive at certain outputs. This lack of clarity can be problematic, especially in high-stakes scenarios like legal or medical advice. A case in point is when an LLM provides a legal recommendation without clear justification, making it difficult for users to understand or trust the basis of that advice. Ensuring that LLMs are transparent and their decision-making processes are understandable is crucial for their responsible use and trustworthiness.
10. Intellectual Property and Plagiarism
LLMs pose challenges in terms of intellectual property and plagiarism. They can generate content that closely mimics existing works, raising questions about originality and copyright infringement. A notable example is when an LLM recreated a passage of text that closely resembled a copyrighted work, leading to legal concerns. Navigating the complexities of intellectual property rights in the context of LLM-generated content is a pressing issue, especially in creative industries where originality is paramount.
What is Large Language Models (LLM)
LLMs are advanced AI systems trained on vast datasets to process and generate human-like text. They are used in various applications, from chatbots and content creation to data analysis and language translation. However, understanding these models goes beyond their technical capabilities; it involves examining their societal, ethical, and practical impacts.
- LLMs are advanced AI models capable of processing and generating text.
- They require significant computational resources and data for training.
- LLMs are used in a wide range of applications across industries.
- Understanding LLMs involves considering their ethical, societal, and practical implications.
- Challenges include bias, accuracy, ethical use, environmental impact, and job displacement.
A real-life example of the impact of LLMs is their use in customer service chatbots, which have transformed how businesses interact with customers, offering 24/7 assistance and raising questions about job displacement and the quality of automated interactions.
Studies on Large Language Models (LLM)
Several studies have been conducted to understand and improve Large Language Models. These studies focus on enhancing accuracy, reducing biases, understanding environmental impacts, and exploring new applications. They provide valuable insights into the capabilities and limitations of LLMs.
- Using large language models in psychology
- This study from Nature Reviews Psychology delves into using LLMs like GPT-4 and Googleโs Bard in psychology. It offers an insightful review of their foundations and discusses LLMs’ transformative potential and challenges in this field.
- How Large Language Models Will Transform Science, Society, and AI
- Stanford HAI’s article examines the broad impact of GPT-3, highlighting its capabilities and limitations, including the generation of biased or factually inaccurate content.
- Large language models encode clinical knowledge
- Featured in Nature, this study introduces the MultiMedQA benchmark for assessing LLMs in clinical contexts. It evaluates models like Googleโs Pathways Language Model, emphasizing their potential and limitations in medical applications.
- Are Large Language Models Ready for Healthcare? A Comparative Study on Clinical Language Understanding
- This research thoroughly evaluates state-of-the-art LLMs for clinical language understanding tasks, proposing novel strategies for healthcare applications.
- A Comprehensive Overview of Large Language Models
- This survey paper from ar5iv.org offers a detailed analysis of LLM architectures, training strategies, and performance evaluations, outlining significant findings and future directions in LLM research.
These studies provide a comprehensive perspective on the advancements, applications, and challenges of large language models in various domains.
Video on Large Language Models (LLM)
Videos on Large Language Models offer a visual and engaging understanding of these complex systems. They range from educational content explaining how LLMs work to discussions on their implications and demonstrations of their applications. Users can explore various online platforms for such videos and may contribute links to insightful videos.
Conclusion
In conclusion, while Large Language Models represent a remarkable leap in artificial intelligence, they are not without their downsides. The concerns, ranging from ethical dilemmas to environmental impacts, highlight the need for careful consideration and responsible use of these technologies. As we continue to integrate LLMs into various aspects of our lives, we must address these challenges, ensuring their development and deployment align with societal values and sustainable practices. The future of LLMs holds great potential, but it also demands vigilance and thoughtful engagement from all stakeholders involved.
Daniel Raymond, a project manager with over 20 years of experience, is the former CEO of a successful software company called Websystems. With a strong background in managing complex projects, he applied his expertise to develop AceProject.com and Bridge24.com, innovative project management tools designed to streamline processes and improve productivity. Throughout his career, Daniel has consistently demonstrated a commitment to excellence and a passion for empowering teams to achieve their goals.