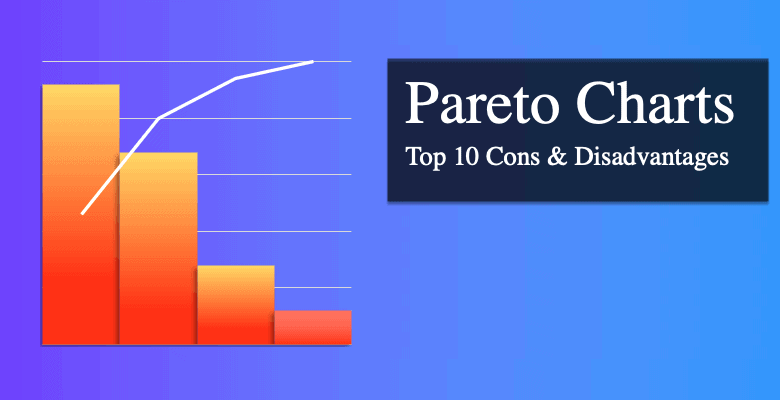
Pareto Charts, named after the Italian economist Vilfredo Pareto, combine bars and a line graph, where individual values are represented in descending order by bars, and the line represents the cumulative total. While Pareto Charts are a powerful tool for highlighting the most significant factors in a dataset and are widely used in quality control, project management, and decision-making processes, they are not without disadvantages.
The Pareto principle underlying these charts posits that a small number of causes often lead to a large portion of the problems, guiding users in focusing their efforts where they can have the most significant impact. However, this focus can sometimes lead to misinterpretation or oversight of critical data. Moreover, the effectiveness of Pareto Charts relies heavily on the accurate collection and categorization of data, making them less suitable in situations where data is incomplete, or categorization is challenging.
Pareto Charts: 10 Drawbacks or Disadvantages
Despite the widespread use and apparent benefits of Pareto Charts in various fields, they come with limitations that can affect their effectiveness and the accuracy of their insights. Recognizing these cons is essential for analysts, project managers, and decision-makers to ensure that the reliance on Pareto Charts does not lead to oversight or misdirected efforts. Below, we delve into the top 10 disadvantages of Pareto Charts, highlighting how they might not always be the optimal tool for every data analysis or problem-solving scenario.
1. Oversimplification of Data
Pareto Charts tend to oversimplify data, presenting it to emphasize certain aspects while potentially neglecting others. This characteristic can be particularly problematic when dealing with complex issues that require a nuanced understanding of various factors. The simplicity of Pareto Charts, while beneficial for clarity and focus, can sometimes lead to a superficial analysis that misses underlying problems or relationships.
- Loss of Detail: Important details and nuances of data can be lost when condensed into a Pareto Chart, which might lead to overlooking subtle yet significant factors.
- Risk of Misinterpretation: The oversimplified representation of data can result in misinterpretation, where critical issues are underestimated or overlooked entirely.
- Inadequate for Complex Problems: Complex problems involving multiple variables and their interactions may not be adequately addressed by the binary nature of Pareto Charts, necessitating more sophisticated analytical tools.
2. Focus on Frequency Over Severity
Pareto Charts prioritize issues based on frequency, which can sometimes overshadow the importance of addressing problems based on their severity or impact. This approach might lead to prioritizing minor issues that occur frequently over more severe but less frequent problems, potentially misguiding resource allocation and efforts.
- Neglect of Critical Issues: Severe issues that occur less frequently may be deprioritized, even if their impact on the system or process is substantial.
- Misallocation of Resources: Resources might be directed towards addressing frequent but less impactful problems, leading to inefficient use of time and effort.
- Skewed Priority Setting: The focus on frequency can skew priorities, leading organizations to address symptoms rather than root causes of significant issues.
3. Limited to Qualitative Data
Pareto Charts are most effective with qualitative data, such as defect types or causes of errors, and less with quantitative data, like measurements or times. This limitation reduces their applicability in situations where the analysis of numerical data is crucial for understanding and solving problems.
- Inapplicability to Quantitative Analysis: Challenges arise when applying Pareto Charts to quantitative data, which may require different statistical analysis methods.
- Limited Scope of Use: The effectiveness of Pareto Charts is confined to scenarios where data can be easily categorized, excluding a broad range of quantitative problems.
- Difficulty in Handling Continuous Data: Continuous, numerical data sets are difficult to categorize effectively for Pareto analysis, often requiring additional preprocessing steps.
4. Assumes 80/20 Distribution
Pareto Charts are based on the 80/20 rule, which assumes that a small percentage of causes are responsible for a large percentage of problems. However, this distribution does not universally apply, leading to potential misinterpretation of data and misguided focus.
- Not Universally Applicable: The 80/20 distribution is an assumption that does not hold for all types of data or situations, potentially leading to incorrect conclusions.
- Risk of Overgeneralization: Relying on the 80/20 rule can result in overgeneralization and neglecting significant factors that do not fit this distribution.
- Misguided Decision Making: Decisions based on the assumed 80/20 distribution may misdirect efforts towards areas that are not as impactful as believed, reducing the effectiveness of interventions.
5. Difficulty in Identifying Complex Relationships
Pareto Charts are not designed to identify or analyze complex relationships between variables, making them less useful for understanding multifaceted problems where factors are interrelated and impact each other in non-linear ways.
- Simplistic Analysis: They provide a simplistic view that may not capture the complexity of specific issues where multiple causes contribute to a problem.
- Lack of Depth in Analysis: The charts do not facilitate the exploration of how different factors influence each other, which is critical for solving complex issues.
- Ineffective for Multivariate Problems: For problems that involve multiple variables and their interactions, Pareto Charts fall short, necessitating more sophisticated analytical tools that can handle multivariate analysis.
6. Static Representation
Pareto Charts offer a static snapshot of data, capturing information at a specific point in time. This characteristic can be a significant limitation in dynamic environments where conditions and data evolve rapidly, making it challenging to use Pareto Charts for ongoing monitoring or for capturing trends over time.
- Limited Use in Dynamic Environments: Pareto Charts can quickly become outdated in settings where data changes frequently, requiring constant updates to remain relevant.
- Inability to Track Changes Over Time: They do not inherently track progress or changes over time, making them less suitable for long-term trend analysis or continuous improvement efforts.
- Need for Regular Updates: Pareto Charts must be regularly updated to maintain accuracy and relevance, which can be resource-intensive in fast-paced or rapidly changing scenarios.
7. Not Suitable for All Types of Data
Certain data types, especially those where issues or categories are equally important or where many categories with similar frequencies, may not be well-suited for representation in a Pareto Chart. This limitation can hinder the chart’s effectiveness and applicability in these contexts.
- Equally Important Issues: In scenarios where all issues need simultaneous attention, Pareto Charts’ focus on prioritizing certain issues can be misleading.
- Large Number of Similar Categories: When many categories exist with similar frequencies, distinguishing between them becomes challenging, reducing the chart’s utility.
- Difficulty with Diverse Data Sets: Pareto Charts may not accurately represent data sets that do not fit neatly into distinct categories, limiting their usefulness in varied analytical contexts.
8. Requires Accurate Categorization
A Pareto Chart is effectiveness heavily depends on the accuracy with which data is categorized. Incorrect or poor categorization can lead to misleading conclusions, as the clarity and insight provided by the chart are directly tied to how well the data is organized.
- Dependence on Data Quality: The utility of Pareto Charts is compromised if the underlying data is inaccurately categorized or of poor quality.
- Potential for Misleading Insights: Inaccurate categorization can lead to incorrect prioritization of issues, potentially misleading decision-making processes.
- Challenges in Data Preparation: Effective use of Pareto Charts requires substantial upfront work in data preparation and categorization, which can be a significant limitation in terms of time and resources.
9. Can Lead to Neglect of Less Frequent Issues
Focusing primarily on the “vital few” causes or issues highlighted by Pareto Charts can result in the neglect of less frequent but still important issues. This approach risks overlooking areas that, while not as prominent in the data, may have significant impacts or may represent emerging trends.
- Overlooked Emerging Trends: Less frequent issues might be early indicators of emerging trends or problems that require attention before they become more significant.
- Neglect of Significant but Infrequent Problems: Infrequent issues with potentially severe consequences might be deprioritized or ignored altogether.
- Incomplete Problem Resolution: By focusing only on the most common issues, organizations might miss the opportunity to address the problems affecting a process or system.
10. Skill and Experience Required
Creating and interpreting Pareto Charts effectively requires a certain level of skill and experience. Without a proper understanding of the chart’s limitations and the nuances of data analysis, there is a risk of incorrect conclusions and misguided decisions.
- Need for Expertise: Effective use of Pareto Charts demands understanding statistical principles and data analysis techniques.
- Risk of Misinterpretation: Inexperienced users may misinterpret the data presented in Pareto Charts, leading to incorrect conclusions and potentially costly mistakes.
- Training and Experience: Organizations must invest in training and developing the analytical skills of their staff to ensure Pareto Charts are used effectively, which can be a barrier to quick implementation.
Conclusion
While widely celebrated for their ability to simplify data analysis and highlight significant issues, Pareto Charts have a range of limitations that can impact their effectiveness in specific contexts. The inclination to streamline complex data excessively, prioritize frequency over impact, and rely on the 80/20 rule can result in misaligning priorities and the oversight of significant yet infrequent problems.
Additionally, their static nature and lack of suitability for all data types further restrict their applicability. Understanding these disadvantages is essential for analysts and decision-makers to ensure that reliance on Pareto Charts does not lead to oversight or misdirected efforts in addressing problems.
Recommended article: Top 10 Cons & Disadvantages of Monte Carlo Analysis
Daniel Raymond, a project manager with over 20 years of experience, is the former CEO of a successful software company called Websystems. With a strong background in managing complex projects, he applied his expertise to develop AceProject.com and Bridge24.com, innovative project management tools designed to streamline processes and improve productivity. Throughout his career, Daniel has consistently demonstrated a commitment to excellence and a passion for empowering teams to achieve their goals.