Top 10 Cons & Disadvantages of Monte Carlo Analysis
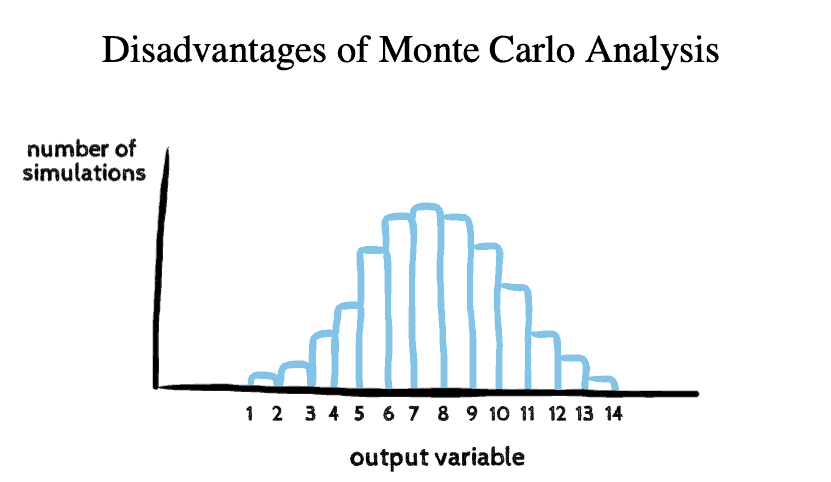
Monte Carlo analysis, a stochastic technique used for modeling the probability of different outcomes in a process that cannot easily be predicted due to the intervention of random variables, has become a staple in various fields such as finance, engineering, and research. Using randomness to solve problems that might be deterministic in principle offers a way to see all the possible outcomes of a decision and assess the impact of risk. However, this innovative approach is not without its drawbacks. This article explores the top 10 disadvantages of Monte Carlo analysis, providing a comprehensive understanding of its limitations.
This technique relies on the law of large numbers, simulating thousands or even millions of scenarios to predict the likelihood of various outcomes. While its applications have been celebrated for bringing more depth and realism into the modeling of complex systems, it’s crucial to acknowledge the inherent challenges and limitations. From computational demands to the quality of input data, understanding these cons is vital for professionals and researchers who rely on these models for critical decision-making.
Top 10 Cons & Disadvantages of Monte Carlo Analysis
The three most significant drawbacks of Monte Carlo analysis are its reliance on high-quality input data, the computational intensity of the method, and the challenge of accurately interpreting results. Each of these aspects fundamentally influences the effectiveness and reliability of the technique. Without high-quality data, the simulations can lead to misleading conclusions. The computational demand makes it less accessible for smaller organizations or projects with limited resources. Moreover, interpreting the results requires a deep understanding of both the method and the modeled system, which can be a significant barrier.
1. Reliance on Quality Input Data
Monte Carlo analysis is heavily reliant on the quality of input data. Garbage in, garbage out – if the input data is flawed, the results will be too. This poses a significant challenge, especially in fields with scarce data or questionable quality. Consider a financial institution using Monte Carlo simulations for risk assessment. If the historical market data they use is incomplete or biased, the simulation will produce risk assessments that are either overly optimistic or pessimistic, leading to poor investment decisions. The institution might either miss lucrative opportunities or expose itself to higher risks than anticipated. This reliance on data quality makes Monte Carlo analysis a double-edged sword, beneficial when the data is good but potentially harmful when it’s not.
2. High Computational Cost
The computational intensity of Monte Carlo analysis is another major drawback. It requires significant processing power and time, especially for complex models with many variables. For example, in climate modeling, simulations must consider countless variables over extended periods. This demands supercomputers and substantial time, making it a resource-intensive process. Smaller organizations or research groups without access to such computational resources may find it challenging to utilize Monte Carlo analysis effectively. This limitation can divide research and application capabilities between organizations with different resource levels.
3. Difficulty in Result Interpretation
Interpreting the results of Monte Carlo analysis can be challenging, particularly for those without a strong statistical background. The method produces a range of possible outcomes and their probabilities, but understanding what these results mean in practical terms requires expertise. For instance, a Monte Carlo simulation might show a 30% chance of project delay in a project management scenario. However, translating this into actionable insights—like whether to allocate more resources or revise project timelines—demands a nuanced understanding of both the project and the analysis method. This complexity can lead to misinterpretation or misuse of the results.
4. Requires Extensive Sampling
Monte Carlo analysis requires extensive sampling to produce accurate results, which can be time-consuming and computationally expensive. For example, engineers must run thousands of simulations to account for various load scenarios and material behaviors when designing a new bridge. This extensive sampling is crucial to ensure safety and reliability, but it also means that the design process can be significantly prolonged, increasing the overall project timeline and cost.
5. Sensitive to Assumptions
The technique is highly sensitive to the assumptions made in the model. The results can be misleading if these assumptions are incorrect or too simplistic. In economics, for instance, if a Monte Carlo simulation of market behavior is based on the assumption that markets are always efficient, it may fail to predict or explain real-world phenomena like bubbles or crashes. This sensitivity to assumptions requires users to be very careful and realistic in setting up their models, which is not always straightforward.
6. Not Always Appropriate for Small Data Sets
Monte Carlo analysis is not always appropriate for small data sets. In medical research, where patient samples for a rare disease might be limited, Monte Carlo simulations can lead to inaccurate or unreliable predictions. The technique works best with large data sets where the randomness can average, but with small samples, the randomness can dominate and skew the results.
7. Can Oversimplify Complex Systems
The method can oversimplify complex systems. In environmental science, for instance, using Monte Carlo simulations to predict the impact of climate change on an ecosystem might not account for all the intricate interactions within the ecosystem. This oversimplification can lead to misunderstanding the system’s dynamics and poor decision-making based on the simulation results.
8. Potential for Misuse
There’s a potential for misuse in the hands of those who do not fully understand the method. Misusing Monte Carlo analysis in risk assessment in the financial sector can lead to underestimating the risks, as seen in the lead-up to the 2008 financial crisis. The technique can contribute to catastrophic outcomes without proper understanding and responsible use.
9. Limited by Model Specifications
Model specifications limit the effectiveness of Monte Carlo analysis. In artificial intelligence, for instance, if the model used in the simulation does not accurately represent the AI’s learning and decision-making processes, the simulation results will not be useful. This limitation places a premium on accurate and comprehensive model development, which can be a complex and challenging task.
10. Difficulty in Quantifying Uncertainties
Finally, quantifying uncertainties in Monte Carlo analysis can be difficult. In pharmaceutical development, accurately quantifying the uncertainty in drug efficacy and side effects through simulations is challenging due to the complexity of human biology and the many variables involved. This difficulty can limit the usefulness of the method in making high-stakes decisions.
What is Monte Carlo Analysis?
Monte Carlo analysis is a computational algorithm that uses random sampling to obtain numerical results. It’s typically used to assess the impact of risk and uncertainty in prediction and modeling applications. The method finds extensive use in various fields, from finance to physics, due to its flexibility and ability to model complex systems with numerous uncertain variables. However, understanding what Monte Carlo analysis entails is crucial for its proper application.
- Random Sampling: At its core, Monte Carlo analysis relies on random sampling to simulate a wide range of possible outcomes in a process.
- Probabilistic Results: It provides probabilistic results rather than deterministic ones, offering a spectrum of possible outcomes and their likelihood.
- Versatility: Its versatility makes it applicable in diverse fields for various problems.
- Complex Systems Modeling: Particularly useful in modeling complex systems where analytical solutions are difficult or impossible.
- Decision-Making Tool: Acts as a powerful tool for decision-making under uncertainty.
A real-life example of Monte Carlo analysis is in project management, where it’s used to predict the probability of completing a project within a certain timeline and budget. By simulating various scenarios, managers can identify potential risks and make more informed decisions.
Monte Carlo Analysis Studies
Several studies have been conducted to explore and validate the effectiveness of Monte Carlo analysis in various fields. These studies aim to understand its applicability, accuracy, and limitations in real-world scenarios.
- Monte Carlo Simulation: History, How it Works, and 4 Key Steps
- Monte Carlo Study by ScienceDirect
- What is Monte Carlo simulation?
- Monte Carlo method
- Monte Carlo Analysis in Academic Research
Monte Carlo Analysis Video
Videos on Monte Carlo analysis offer visual and practical insights into how the method works and its applications. These can range from academic lectures to practical examples in industry settings. Users can supplement their understanding by watching these videos, which often break down complex concepts into more digestible content.
Conclusion
While widely recognized for handling uncertainty in diverse sectors, the efficacy of Monte Carlo analysis is not without its significant constraints. Paramount among these is the critical dependence on the quality of input data; inaccurate or biased data sets inevitably lead to flawed conclusions, rendering the analysis ineffective or even misleading. This necessitates an unwavering focus on meticulous data collection and validation, a step that cannot be compromised.
Additionally, the computational demands of Monte Carlo simulations pose a substantial barrier, especially for smaller organizations or research endeavors with limited resources. This creates a divide in the practical applicability of this method and highlights the urgent need for advancements in computational technologies and algorithms. Such developments could democratize access to this potent analytical tool, extending its benefits beyond the confines of well-resourced entities.
Recommended article: Top 10 Cons & Disadvantages of Poisson Distribution